What is the significance of generative artificial intelligence for the manufacturing industry? In this discussion, Swisscom experts Michael Rieger and Hanspeter Groth explore the opportunities and limitations and suggest entry points for companies.
Brief summary of the discussion
In this comprehensive interview, Michael Rieger (left in the image accompanying the article) and Hanspeter Groth (pictured on the right) discuss potential applications and effects of generative AI in the manufacturing industry. By independently revealing new content and solutions, generative AI opens up opportunities for creative and innovative approaches.
The benefits of generative AI in the manufacturing industry are manifold – from chatbots that provide specific assembly instructions to improved forecasting and HR support – and may lead to better product quality and efficiency.
But despite the potential for automation and efficiency gains, human expertise remains crucial. This applies in particular to the interpretation of the results delivered by AI and the successful implementation of AI projects. A holistic strategy and the right governance are therefore vital.
About the experts
Hanspeter Groth, an engineer with many years of experience in the manufacturing industry, is Industry Leader for Manufacturing at Swisscom.
Michael Rieger is Technical Strategist at Swisscom’s CTO office, where he assesses the impact of new technologies on the telecommunications and ICT industry.
Digital Manufacturing Services
Industry leaders have increased their productivity by up to 90% through digital transformation. Swisscom specialists support you in the digitalisation of production.
Everybody’s talking about artificial intelligence right now, especially generative AI. What’s the situation in the manufacturing industry?
Hanspeter Groth: AI is not a new topic. I don’t know of any industrial company that is not using AI. But that is traditional AI – for dynamic adjustment of inventory levels according to needs and demands and for forecasting, for example. Retailers, for instance, are taking weather forecasts into account when planning how much ice cream to stock in their stores. This involves the use of AI models that the companies have trained themselves and integrated into their own processes.
Generative AI is now the next step. I’m interested in how this will be different.
Michael Rieger: There’s a good way to explain the difference. As you mentioned, companies have been using traditional AI to make forecasts based on existing data patterns. What sets generative AI apart is its ability to independently generate new content, rather than just automating predefined tasks or reacting to previous data. This means that generative AI can support human creativity by providing ideas, taking on routine tasks and speeding up creative processes, all things which are only possible to a limited extent with traditional AI.
Groth: In a planning scenario, that would mean not only using internal data, but other data as well? I might analyse competitors and market trends and generate completely new forecasts that would not have been easily possible with traditional AI in this form.
But that still requires that I can access the data I need and also trust it. In order to train traditional AI models, I have to prepare and structure my data precisely. Is that no longer necessary for generative AI?
Rieger: Generative AI certainly benefits from structured data, but it can also work effectively with unstructured data. In fact, generative AI models are capable of gaining valuable insights from a variety of data formats. As a somewhat basic example, ChatGPT has been fed content from the internet without the data having been strictly structured. The result is a model that can cover a multitude of languages and, above all, topics that any one individual would probably not be able to cover.
This shows that generative AI can deliver good results even when using unstructured data. Companies can benefit enormously from this difference, because most data is available in an unstructured form. New data, such as social media posts, can be incorporated directly without having to be structured first. Generative AI therefore handles information in a similar way to us humans.
How does generative AI work in practice?
Groth: Take the following use case. A company produces hundreds of products that are all very similar to each other. This means that the tasks that assembly employees have to perform change frequently. All assembly instructions are available in text form, which is not particularly well structured. Until now, employees have had to look for the right instructions or ask the foreman.
Generative AI could be used to train a chatbot with this information so that employees could ask a question and get high-quality answers. What do you think, Michael?
Rieger: Exactly, that’s a good example. Generative AI models are already pre-trained and have an astonishingly good command of human language. These AI models can also be enriched with specific in-house knowledge, in this case the assembly instructions. Employees can query this knowledge in natural language via a chatbot, which can deliver good results if the data is good – even if some of the data is only available in an unstructured format.
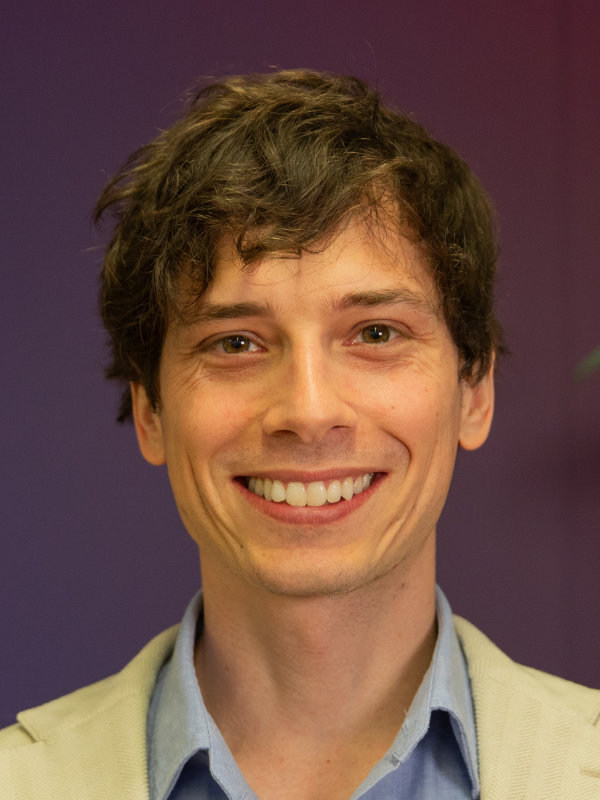
‘Generative AI models are capable of gaining valuable insights from a variety of data formats.’
Michael Rieger, Technical Strategist at Swisscom’s CTO office
This is true in the manufacturing industry, but is also very important in all industries. Take, for example, customer service, where there is a lot of unstructured data from e-mails, calls and so on. This information can be used directly by generative AI without having to be structured first.
Groth: Returning to the topic of forecasting, there’s a further takeaway for me. In the past, I knew roughly which customer buys how much in what time. In manufacturing, for example, we have traditionally created forecasts over the last 20 years. Now I can go a step further and add competitor analysis with unstructured data to my forecast. I can look at what’s going on in the market and what I find on the internet and in annual reports – what my competitors’ sales are like, what they are planning and where they are investing, what my customers are concerned about and what trends might have an impact. I can now gather all this information and use it with generative AI.
Of course, someone still has to think about whether the information can really be trusted, as I touched on before. But generative AI can make me more efficient and better at competitor analysis.
Rieger: Exactly, generative AI provides support where information from different sources needs to be collated and interpreted. Perhaps I would like to put the geopolitical situation in perspective and find out whether a company is therefore a good supplier or I could potentially face a bottleneck. Generative AI can process this data, recognise the connection between the geopolitical situation and my supplier and capture that as a reference point. This gives me the opportunity to consider this aspect as well if I was not already aware of it.
What impact will generative AI have on employees’ work?
Groth: For me, this also means that I need more employees who can think in terms of contexts and pose critical questions. Generative AI is then extremely valuable. But it’s not something that everyone can easily apply without prior knowledge. I’m not talking here about improving a text with Copilot. I mean using generative AI to optimise processes, improve forecasts or find the right job applicant. Or how do you see this?
Rieger: I agree with you. Generative AI, like traditional AI already, offers employees a way to delegate certain tasks. The results I get back from generative AI must then be checked for quality by employees. For this, I still need competent people who are able to critically scrutinise the information.
Groth: So it’s important for companies to have a handle on a lot more than just technology – on grounds of security, for governance reasons and also because of employees. Generative AI is sure to change how some jobs are done. For example, a new task may be checking the output of generative AI.
Rieger: Yes, it’s very important that the entire organisation takes this step. In manufacturing, we’ve seen new technology initially having a purely supportive function for blue-collar employees. Over time, however, the role of assembly employees has changed. Generative AI is also changing the work of white-collar employees. Companies have to be careful here. After all, there are – understandably – many people who see AI less as an efficiency aid and more as a threat to their jobs.
In which areas could manufacturing companies use generative AI?
Groth: AI is likely to bring benefits in almost all areas of manufacturing. Let’s start with interacting with customers. For example, companies need to understand, interpret, correctly channel and learn from service interactions to make their service offering better, faster and more efficient.
ERP systems such as SAP are often in use in the areas of planning, logistics and finance. Here, too, there are ways to become more efficient. Because the data is highly structured, that might involve the use of more of a traditional AI to identify patterns of which customers buy when and why. You could definitely think of this in terms of low-hanging fruit.
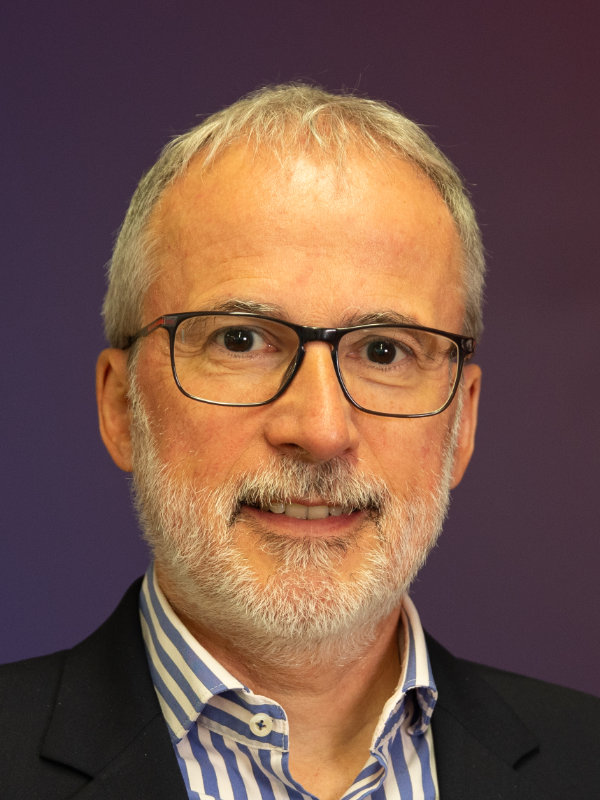
‘There are extremely exciting use cases for generative AI in manufacturing companies.’
Hanspeter Groth, Industry Leader for Manufacturing at Swisscom
I could also imagine efficiency improvements in sales. Mechanical engineering firms will always have to provide complex quotes. If I use generative AI, it could produce for me an individual draft of a new quote based on existing quotes. Of course, this wouldn’t mean the quote is ready, as I’d still have to check it and add to it. But it would help me to take into account all the elements I need and maybe even reuse some elements on a one-to-one basis. These are extremely exciting use cases for companies.
Why should manufacturing companies even use AI?
Groth: Companies are moving away from selling just machines – instead, the services associated with them are becoming increasingly important. Ultimately, it’s about ensuring customers are able to use the machines and products efficiently and effectively in their operations. AI can help with this, for example with predictive maintenance or by advising customers on how to optimise production. Companies can also use AI to determine which shifts and employees are highly efficient and which are not.
Even if I can’t quite figure out the business case with AI – if I don’t do it, then someone else will. And then maybe at some point I won’t have a business anymore, because I can’t keep up with my products and services. So you have to find a way to keep pace with future developments.
Rieger: What you’ve said is very important. Customers have certain requirements as to how they want to obtain services from providers, whether in manufacturing or any other industry. If an artificial assistant is available around the clock to help customers select products and to make the purchase process easier, this could be a USP for the company in question – which might also help it to gain a market advantage.
Groth: The Swiss model of success is usually not about competing to be the cheapest. Instead, we deliver the best in our niche – the best customer experience, a superior machine that best meets customer requirements, with the necessary service.
That means I have to keep working at this or I’ll get overtaken. I have to think about how these technologies fit in my company. Then I will remain competitive in my niche, because otherwise I will be left behind.
Rieger: Companies need to be careful that they do not get left behind as the technology advances. Instead of trying to be the first to apply generative AI to all areas of their business, they should focus on the solution that brings them the greatest benefit and then implement that. They can then evaluate what has been achieved and what it means for the business case: is this something I have to subsidise or is it self-supporting? Is it something that my customers simply expect of me?
In the manufacturing environment, it seems to me that there has been major disruption to supply chains in recent years. What do you think will be the impact of generative AI on the supply chain? This is one of the biggest areas in my view.
Groth: That’s a complex one. Could generative AI have predicted bottlenecks such as those caused by the war in Ukraine, for example? I’m not so sure. On the other hand, if I have more data, I can better plan when and where I need which material. Unfortunately, along the supply chain, it’s not unusual to find that you rarely have exactly the right level of inventory and often there’s too much or too little in stock, despite meticulous planning in respect of raw materials, intermediate products and also end products. If we now manage to combine highly structured knowledge from planning software with additional knowledge from generative AI, it will help us to improve in this area. It should be possible to reduce inventories by 1 to 2 percent, which represents pure profit. Then you probably wouldn’t even have to do the sums for the project, it would pay off immediately. If planning accuracy in the supply chain can be increased through early identification of what is needed, there is great potential there.
What does the situation look like on the cost side?
Groth: Cost-benefit analysis is already commonplace in traditional AI projects. Less so in the case of generative AI, as I can at best assess only certain efficiency gains in monetary terms. But developing a complete business case is likely to be extremely difficult. Entrepreneurs often think differently, they invest in something new because they believe in it. If you could simply calculate the outcome every time, then everyone would do it. Fortune favours the brave, and this should also apply to the use of AI.
Rieger: Precisely because generative AI has created a completely new form of cost assessment, the costs are difficult to calculate. This is one of the reasons why I recommend starting with small projects. Then you’ll learn. But if you start with the biggest project and you lack knowledge and expertise, you probably won’t end up with a positive business case.
If companies are interested in using generative AI, how should they go about this?
Groth: Take Microsoft Copilot, for example – can I simply let my employees loose with this or might this pose a data security risk, considering governance, etc.?
It’s presumably a completely different case if I want to integrate generative AI applications into business processes, as in the example above of the company that wants to improve its forecasting using information from the internet. I suppose I need a holistic strategy for that?
Rieger: Yes, I would definitely recommend a strategy that suits the company. But you must also ensure data quality. On the one hand, you need data to get started. And on the other hand, you need someone who is comfortable using the technology. Technical expertise is required to assess which data is useful and in what form in order to obtain results of the desired quality. And, of course, you need an understanding of security, of which data you want to make available to the AI model. You probably don’t want your chatbot sharing sensitive data with employees or customers.
Past experience has also shown that many projects in the traditional AI environment are already failing because of the data issue. Companies may have lots of ideas and ambition, but problems often arise because they do not have enough data to train their AI model to ultimately deliver the desired results.
Finally, a quick look into the future: in what direction could generative AI develop?
Groth: If you’ve already designed thousands of similar products, then as a company you tend to always think in the same way and usually it isn’t obvious to you that you could do something fundamentally different. If the models become so clever that they can see when you’re trapped in a pattern, they might be able to help you to break out of it. But I think that’s going to take a long time.
And I have trouble imagining that generative AI will ever be as creative as, say, the inventor of the internal combustion engine.
Rieger: I don’t entirely agree with you. I read recently that generative AI is already very good at understanding empathy and responding to people. Even if it won’t be as creative as the engineers who contributed to the development of the internal combustion engine by tomorrow or the day after, the technology is still evolving rapidly. It will be exciting to see where we’ll be in a year’s time.
White paper: Generative artificial intelligence
The market is currently abuzz with news and breakthroughs in the field of generative AI. In view of this dynamic, businesses face the question of how to utilise AI to make their business models future-proof, successful and efficient.
However, what is generative AI and how will it affect businesses in future? What are the possible applications for it? What are the opportunities and what risks do companies need to be aware of? Our white paper provides detailed answers to all of these and many other questions.
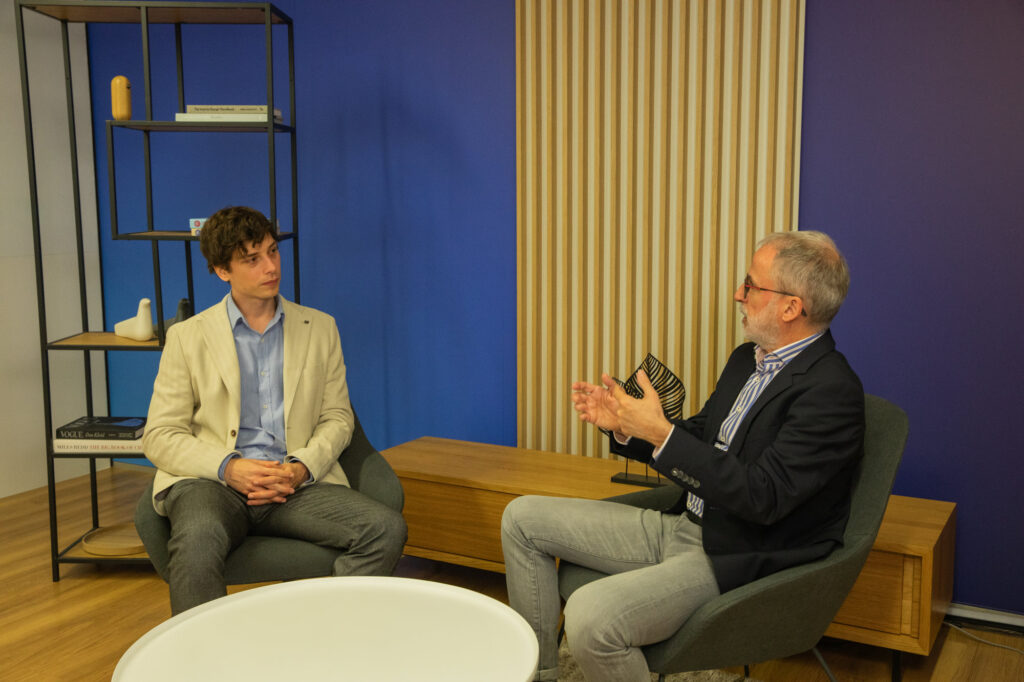